We’re doing cutting edge research for transparent, auditable AI alignment
- Current methods of “alignment” are insufficient;
evaluations are even worse. - Human intent reflects a rich tapestry of preferences, collapsed by uniform models.
- AI`s potential hinges on trust, from interpretable data to every layer built upon it.
- Informed decisions around risk are not binary.
- Training on raw human data doesn’t scale.
- Your models should adapt and scale, automatically.
Solving the most pressing problems in AI
Democratizing AI research is essential, as the future of transformative technologies should not be confined to the corridors of a few profit-driven entities, but open to independent inquiry and understanding for the collective good.
- Fully auditable, robust AGI alignment platform
- Pre-training scale automated dataset curation and augmentation
- Collaborate with top research schools & global community
- Build scalable supervision for agentic workflows
- Work on dynamic and continual RLAIF
- Make multi-modal agents safer
It's time to build
Louis Castricato, Nathan Lile, Suraj Anand, Hailey Schoelkopf, Siddharth Verma, and Stella Biderman
Existing methods for controlling language models, such as RLHF and Constitutional AI, involve determining which LLM behaviors are desirable and training them into a language model. However, in many cases, it is desirable for LLMs to be controllable at inference time, so that they can be used in multiple contexts with diverse needs. We illustrate this with the Pink Elephant Problem: instructing an LLM to avoid discussing a certain entity (a “Pink Elephant”), and instead discuss a preferred entity (“Grey Elephant”).
Supported By
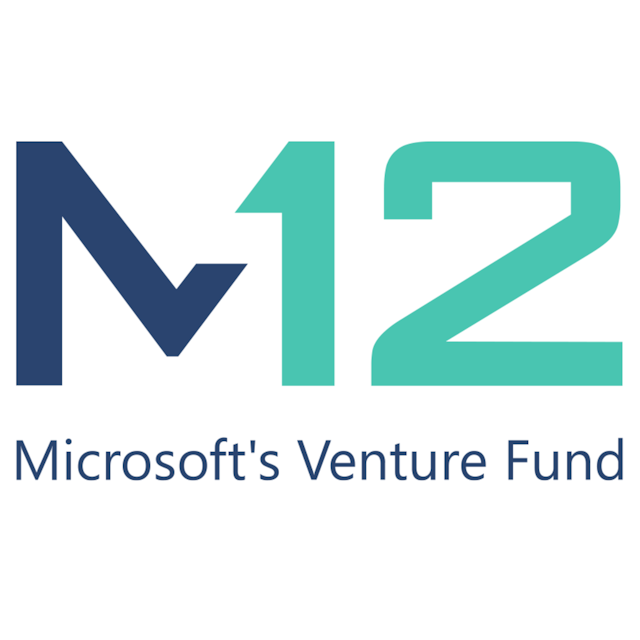
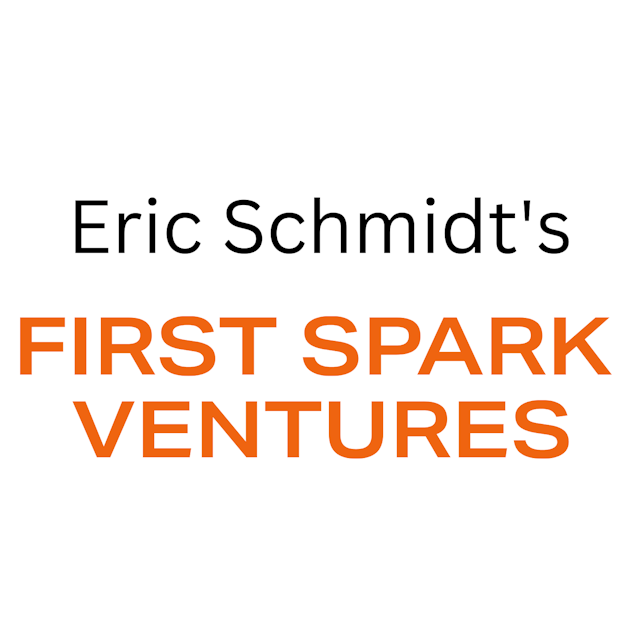